How Do Machine Learning Models Help Businesses in Customer Lifetime Value (CLV)?
- saurabhkamal14
- Feb 4
- 2 min read
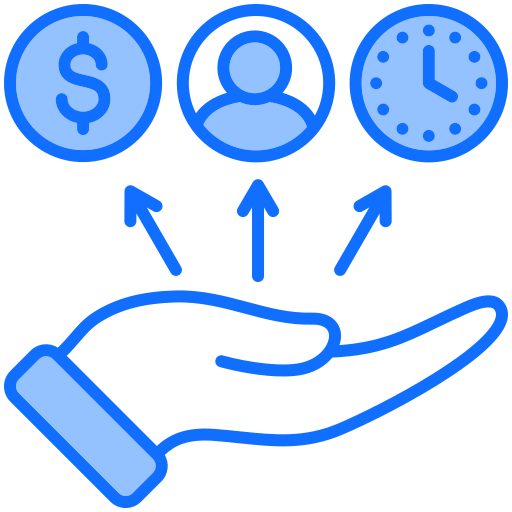
Machine learning (ML) models play a crucial role in helping businesses predict and maximize Customer Lifetime Value (CLV). CLV is a measure of how much revenue a customer is expected to bring to a business over their lifetime as a paying customer. Here’s how ML models assist in this process.
1. Accurate CLV Predictions
Machine learning models analyze large volumes of customer data to identify patterns and predict:
How much a customer is likely to spend.
How long a customer is likely to stay loyal.
Example:
A digital bank can use ML to predict the lifetime value of a new customer based on:
Their initial deposit amount.
How frequently they use the bank's services (e.g., bill payments or loans). For instance, customers who frequently use credit cards or apply for loans might have a higher CLV due to associated fees and interest payments.
2. Identifying High-Value Customers
ML models help fintech companies distinguish between high-value and low-value customers by analyzing spending and engagement patterns.
Why this is important: Fintech companies can focus their marketing efforts on customers who are likely to generate the most revenue, rather than spending resources on less profitable segments.
Example :
A wealth management platform can use ML to identify customers with high investable assets and consistent investment behavior. These high-value customers can then be offered premium advisory services or exclusive financial products.
3. Personalized Marketing
ML models enable fintech companies to create personalized marketing campaigns based on a customer’s predicted CLV.
How this works: ML models analyze customer preferences, transaction history, and behaviors to recommend relevant financial products or services.
Example :
A payment app can send personalized promotions:
High-value customers might receive cashback offers on large transactions.
Lower-value customers might be encouraged to use specific features (e.g., recurring payments) to increase engagement.
4. Optimizing Customer Retention
Retention is a key driver of CLV, and ML models can predict when customers are at risk of leaving (churning).
How it helps:
Fintech companies can take proactive measures, like offering rewards or personalized financial incentives, to retain valuable customers.
Example:
A lending platform can use ML to identify borrowers who might not renew their loans and send them offers with lower interest rates or extended payment plans.
5. Improving Budget Allocation
ML models help fintech companies allocate their marketing budgets more efficiently by predicting the ROI of different campaigns and strategies.
How this works:
Focus on acquiring customers with high predicted CLV.
Avoid wasting budgets on campaigns targeting low-value or churn-prone customers.
Example:
A neobank can use ML to identify that social media ads targeting young professionals yield high-value account holders, whereas campaigns targeting college students may have lower returns. This allows the bank to focus its resources on the more profitable segment.
Conclusion
Machine learning models transform raw customer data into valuable insights for fintech companies, enabling them to predict, grow, and optimize Customer Lifetime Value. By leveraging these models, fintech companies can focus on high-value customers, personalize experiences, reduce churn, and drive long-term growth.